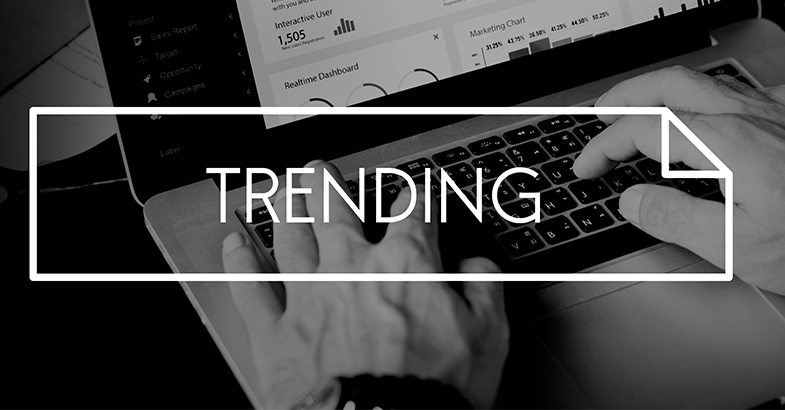
With increased digital adoption and growth in e-commerce, the amount of data generated by the retail industry is enormous. In the past, brick-and-mortar stores could track footfalls and purchases per customer. Now, new information sources such as browsing histories, click rates, explicit feedback in likes, comments, and reviews on social media are omnipresent. This information is helpful for the Fashion industry to keep track of the consumers’ tastes & preferences, demands, and sentiments. As a result of such widespread availability of Big Data and the rapid development of Machine Learning and Data Analysis, companies, and businesses in the Fashion Industry are experiencing a data revolution impacting their day-to-day management decisions. Companies need to extract valuable insights from the data to make well-informed business decisions in this highly competitive industry.
It is critical that a fashion retailer keeps track of the current fashion trends and implements the same in its manufacturing and marketing-related decisions. All this while simultaneously cutting inventory costs. Fashion Forecasting can help fashion retailers to stay abreast with the industry’s transformational shifts, from sustainability targets to changing consumer lifestyles to digital design. With the surge of the large quantity of unstructured data, it has become increasingly important to design and develop a methodology that enables fashion outlets to keep an eye on the ever-changing fashion circuit.
During the Pandemic, the textile industry had slumped to $75 billion after peaking at $106 billion in FY-2020.2 (https://www.businesstoday.in/latest/economy/story/road-to-recovery-textile-industry-pegged-to-grow-300-over-next-2-years-despite-covid-impact-says-report-311923-2021-11-11). The Pandemic-related disruptions muted the domestic demand and exports were lower than expected numbers too, leading to a substantial unsold inventory.
As time elapses, the consumer’s taste & preference also changes due to fast-changing fashion. Any company stuck with large unsold inventory is forced to offer significant discounts to clear old stocks, adding to the existing lower business performance (resulting from lower sales). Predicting fashion trends in time can increase sales and reduce inventory.
This study designed and developed machine learning models that automate the forecasting process based on fashion trends. They used different data sources to train the model and for prediction purposes.
Researchers used an open-source database to train the model. The presence of several open-source databases made the model’s training more effortless. For prediction and training of the model, researchers extracted as many trending, fashion-forward style images as possible from social media sites, blog posts, e-commerce platforms, etc., by targeting fashion influencers, using the main fashion-based keywords and hashtags. The team used the ‘Large-scale Fashion (DeepFashion) Database’ to extract data to train the model. The database used had over Eight hundred thousand images, richly annotated with massive landmarks. The database enabled facilitating powerful algorithms in clothes recognition.
Clothes and fashion have visual appeal. Hence, successful implementation of the project required image analysis. This study converted images into embeddings using feature extraction to extract new features. A faster model that consumed less computational speed was adopted for Transfer learning. The team retrieved Image embeddings and applied the model for an effective recommendation.
Overall, The ML model was successfully implemented using image embeddings, transfer learning, and applying the nearest neighbour algorithm. Integrating both texts & Images ensured convenience for the user to search for pictures either by a search word or via images. Researchers created a simple, easy-to-use User Interface (UI) that even a non-technical person could use.
As Deep Learning is ever-evolving; the study recommended automation or frequent revaluation and recalibration of the model for periodic data extraction.
References:
1: Nagadevara, Singireddy S, ‘Application of Fashion Trend Tracking in a Data Model Case Study,’ 2021